S
Subhash Kak
Guest
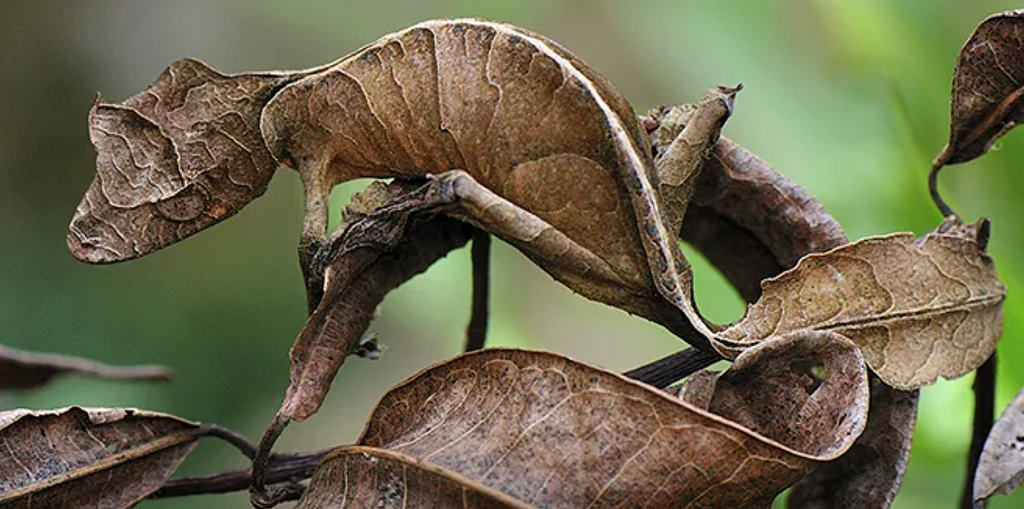
Gecko amongst leaves
Biological systems look somewhat similar across scale and sometimes so even across species. In the picture above, it is difficult to separate the gecko from the leaves.
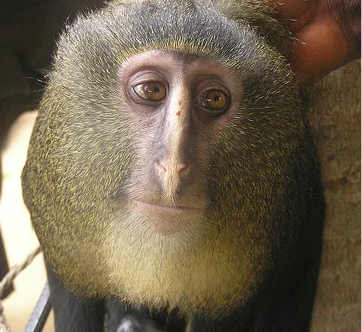
Lesula monkey
Doesn’t this clear-eyed Lesula monkey look like a dean at a university, or perhaps a philosopher?
The similarity across scale is in physical structures and in the way networks are formed and data is exchanged within the system and clearly that ought to be a consequence of the natural laws that underly phenomena. Natural systems have self-similar properties which is how we have spiral structures in plants as well as in the very shape of galaxies [1]. Another example is that of the human inner ear (cochlea) that looks like a snail.
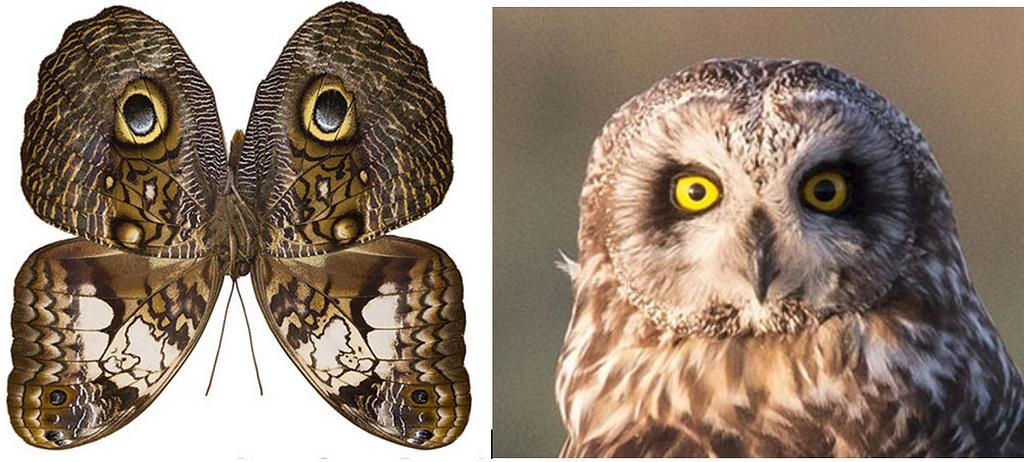
Owl butterfly (left) and an owl (right)
Nature also has mimicry as in the leaf insect below that looks like a leaf. Mimicry is when two or more organisms who are not closely related resemble each other, which improves the survivability of one or both species. In Batesian mimicry, a harmless organism looks like a dangerous one, deterring potential predators or competitors. In aggressive mimicry, an animal looks like prey or a harmless species in order to lure an unsuspecting victim in.
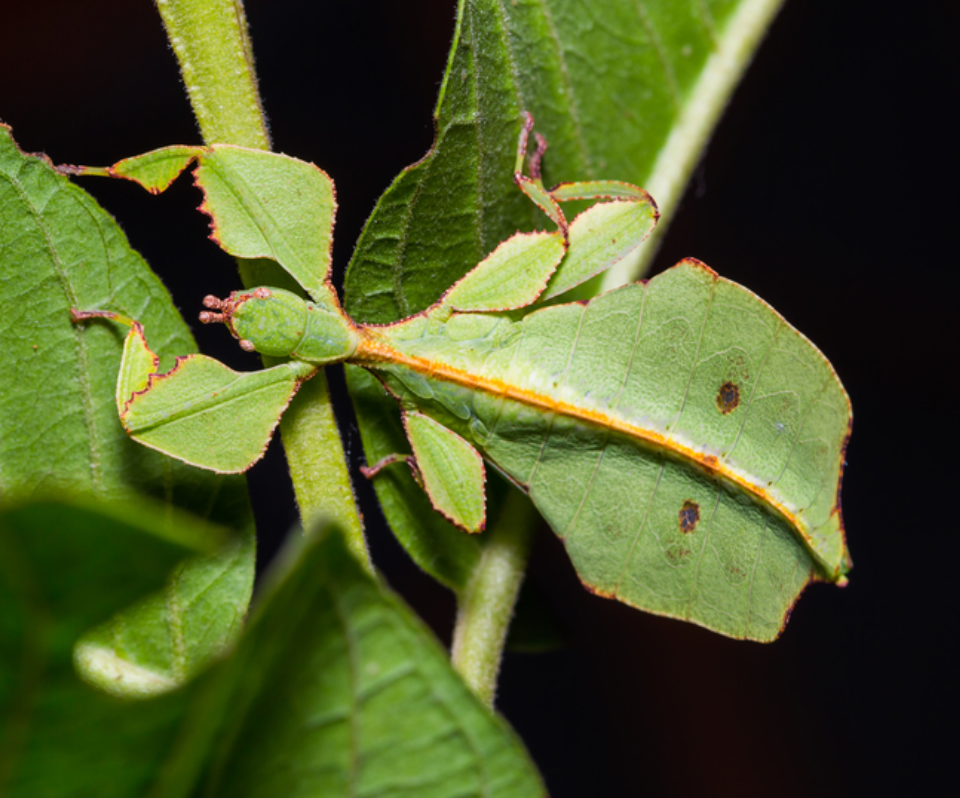
Leaf insect
In the case of the brain, the scales relate to physical geometry as in the arborization of dendrites and axons at the microscopic level, connectivity patterns of networks at the middle (mesoscopic) level, and the scale-free dynamics at the macroscopic level in the long-range, inter-area connecting fibers.
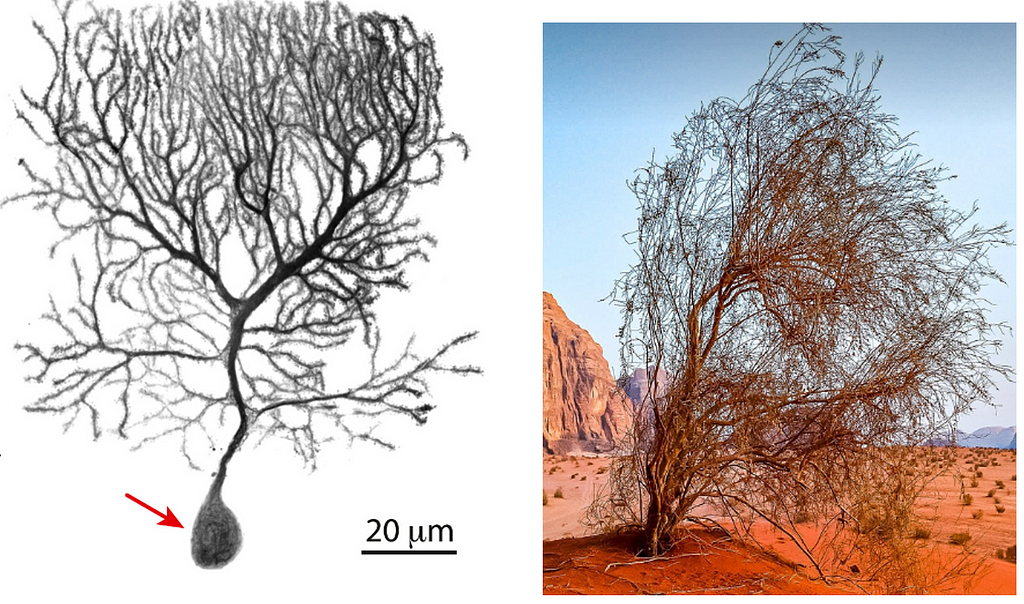
Neural dendrite arborization (left); desert shrub (right)
The geometries of microscopic and macroscopic arboreal structures are reasonably well mapped out, but the logic of the middle-level (mesoscopic) connectivity is yet to be deciphered. In other words, scholars have been unable to explain why the nature of structures at the microscopic and macroscopic levels leads to the emergence of self-similar networks at the intermediate, mesoscopic scale.
Is the principle of scale-freeness, which appears ubiquitous at all levels of structural and functional organization of the brain the unified understanding that links the different scales?
I have previously discussed the application of e-dimensionality (required for optimality) to physical information in a series of papers. (For a quick summary with list of published papers, see [1] that shows how it solves many significant problems such as that of the missing dark matter and dark energy.)
If reality is e-dimensional, which means it is non-integral, then mathematics requires the space to be recursive and scale-free. In popular language it leads to fractal behavior.
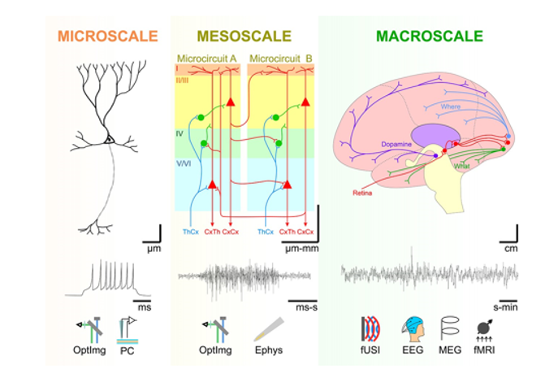
Scalng levels in the brain [2]
Here we provide the logic behind scale-freeness. Since nature is optimal, we should have evidence of e-dimensionality also for biological systems. We have shown that it helps solve five fundamental problems:
1. Explanation of the count of twenty amino acids in the genetic code [3];
2. Shows why the number of codons that map to amino acids are non-uniformly distributed [4];
3. Explains the experimentally observed fractal dimension of chromatin [5].
4. Explains emergence of α-helices and β sheets [6].
5. Explains the emergence of fractal like data [7].
Optimal information representation should also be true for neural systems. We have applied this to get interesting insights into the number of independent cognitive centers in the brain [8]. Somewhat related are theorems on why machines will never be conscious [9], and the relationship of power laws that characterize biological signals to number-theoretic partitions [10] that were investigated by Srinivasa Ramanujan over a century ago.
References:
1. Our e-dimensional universe.
2. George F. Grosu, Alexander V. Hopp, Vasile V. Moca, et al., The fractal brain: scale-invariance in structure and dynamics, Cerebral Cortex 33, 4574–4605, 2023; https://doi.org/10.1093/cercor/bhac363
3. S. Kak, The dimensionality of genetic information, Parallel Processing Letters, vol. 33 (2023); https://doi.org/10.1142/S0129626423400121
4. S. Kak, Self similarity and the maximum entropy principle in the genetic code. Theory in Biosciences 142, 205- 210 (2023); https://doi.org/10.1007/s12064-023-00396-y
5. S. Kak, The geometry of chromatin, TechRxiv (2023); https://doi.org/10.36227/techrxiv.23818902.v1
6. S. Kak, Threads and spirals in a noninteger dimensional universe. TechRxiv. (2023); https://doi.org/10.36227/techrxiv.24112434.v1
7. S. Kak, The iterated Newcomb-Benford distribution for structured systems. Int. J. Appl. Comput. Math 8, 51 (2022); https://doi.org/10.1007/s40819-022-01251-2
8. S. Kak, Number of autonomous cognitive agents in a neural network. Journal of Artificial Intelligence and Consciousness 9, 227- 240 (2022); https://doi.org/10.1142/S2705078522500023
9. S. Kak, No-go theorems on machine consciousness. Journal of Artificial Intelligence and Consciousness 2023; https://doi.org/10.1142/S2705078523500029
10. S. Kak, An information principle based on partitions for cognitive data. Journal of Artificial Intelligence and Consciousness, 10, 1–14, 2023; https://doi.org/10.1142/S2705078522500138
Continue reading...